To demonstrate the model's effectiveness to investors and gather real-world feedback, we developed a proof of concept focused on the greater Seattle area. This allowed us to test the technology with realtors, home owners, and real estate investors in a controlled environment.

Giving users visibility into why a home has a specific value
Unlike opaque online valuations, we wanted to offer transparency. We went beyond just a number; we empower our customers with insights into the specific features that influence a home's value, both positively and negatively.
How much is this home worth right now?
Users don’t need to know how much a home’s value was two months ago. Traditional home valuation services often rely on outdated data, providing estimates that can be months old. We wanted to show real-time values, keeping users abreast of ever-changing markets and current values.


“Explainability”: simplifying complex financial concepts, like equity and appreciation
More early feedback revealed a common challenge: homeowners struggled to grasp many financial concepts related to their property. To address this, we developed data-driven visualizations that make equity, appreciation, and other financial aspects of homeownership clear and accessible.
Journey maps bridge the gap for our product and dev teams, aligning everyone on Amy’s experience. They also highlight key opportunities where Plunk can best support her needs.
To help set a realistic prioritization (we are a startup after all) we group user stories and feature suggestions around user journey phases, and then attach “level of effort” and “value to user” to each idea.

It was a lot easier to find concepts that tested well and were useful to our users. But choosing a delivery method that satisfied everyone? That proved trickier. Some users wanted a mobile or web app, while others needed lead-generation widgets they could embed on their sites. Other, more sophisticated customers just wanted the data. Delivering a solution that satisfied everyone’s needs presented its own challenges, but it was necessary to make sales. So we started with individual APIs as the foundation for a multitiered deployment.
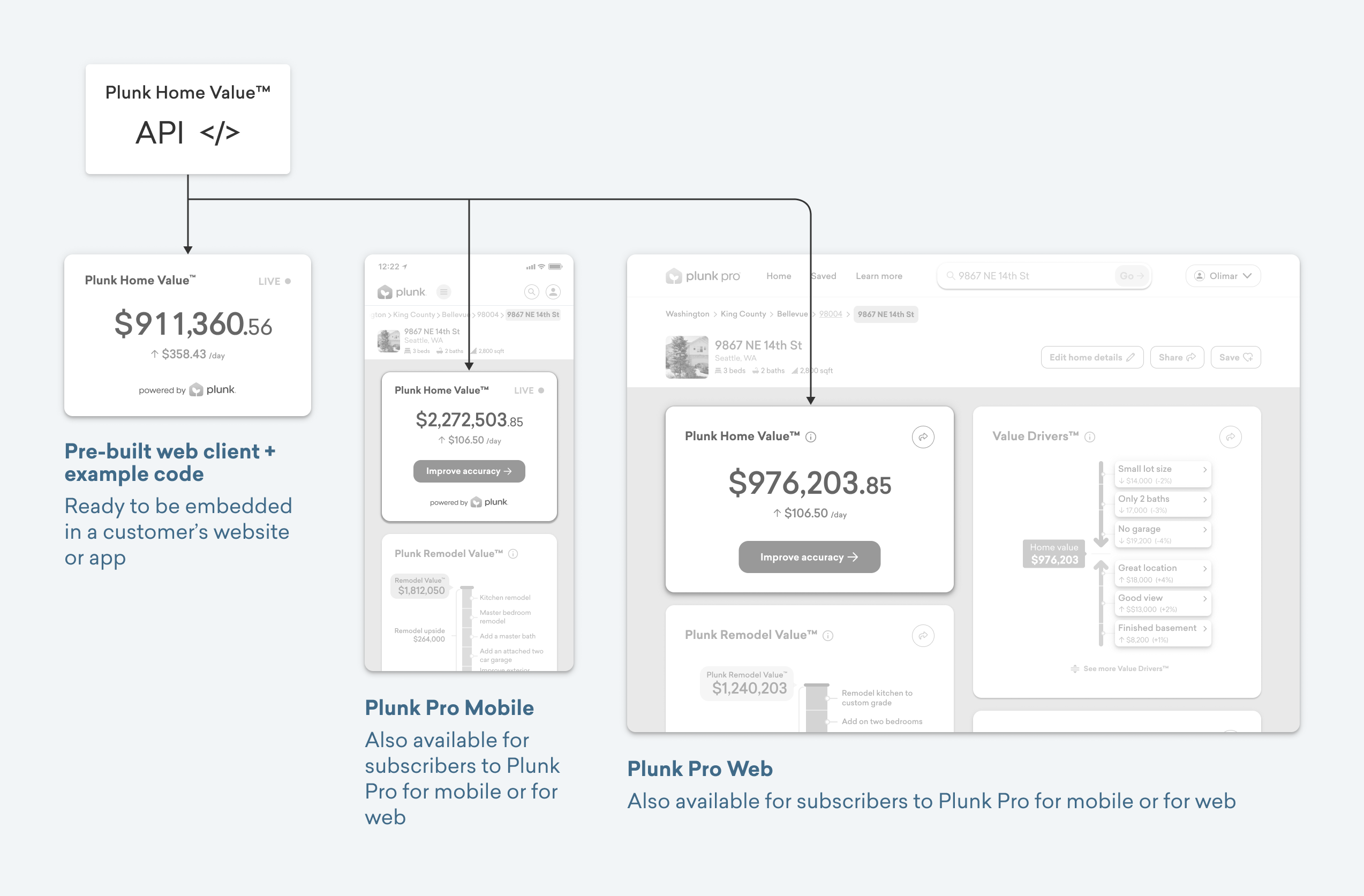
As with any project, the more stories you hear from your customers, the more your assumptions fall away and the clearer your path forward is. Here are some of our main takeaways:
This is the very reason why real estate professionals consistently harbor mistrust towards companies like Zillow and Redfin. Realtors possess an intimate understanding of homes and the markets they inhabit. Time and again, we've heard from realtors expressing skepticism towards online real estate platforms, citing inaccurate valuations and reliance on outdated data. Viewed not as an ally, but as a hindrance. This sentiment guided our product development journey: how can we empower realtors to truly shine as experts in their field?
One of our first imagined scenarios was that realtors would use our analytics to find hidden gems, or discover trends in local real estate markets that most people couldn't see. It turns out they don't need much help in that arena. What they do struggle with, though, is explaining to their clients why a home is a gem, or being able to describe trends in local markets. What realtors really need from us is backing for their arguments, home and market data, and data visualizations that help demonstrate to their clients that their expertise and advice is spot on.
We tested prototypes that allowed realtors to make fine-tuned adjustments to the home valuations, and alter the adjustments made when comparing two homes. We heard a pretty consistent theme: "Wow, this is great. But I usually skip this part. It just takes too long". Everyone we tested agreed that these adjustments were very important, but the universal opinion after testing (even from our most technically savvy realtors) was, "Yes this is important, but just do it for me. I trust you to do it better than I will."